Spatial Biology and Computational AI for the future
- gandhamkumarsatyar
- Apr 1, 2023
- 4 min read
In recent years, spatial biology tools have swept the field of life sciences and pharmaceutical research. Pathologists, who examine tissue sections for clinical diagnosis are aware of the value of spatial context in tumorigenesis and metastasis. This new set of tools has the potential to be revolutionary in the field of medicine and life sciences as it has transformed there search methodology. To
combine the best of both worlds, these tools deliver in-situ deep molecular profiles from a tissue section.

Imagine the knowledge you would typically gain from a histology slide, and how much more valuable it would be to add a layer of in-depth understanding about the expression of dozens of genes or proteins at single-cell and even sub-cellular resolution to reveal crucial information about cellular and molecular interactions.
Amazing discoveries about the function of immune cells in the tumour microenvironment, the distribution of viral particles in cases of infectious disease, and the progression of neurodegenerative diseases have already been made, thanks to spatial biology. Even though these discoveries originated in research labs, pathologists and clinical researchers are already applying them to derive insights from tissues.
Pathology Labs: Revolution in progress
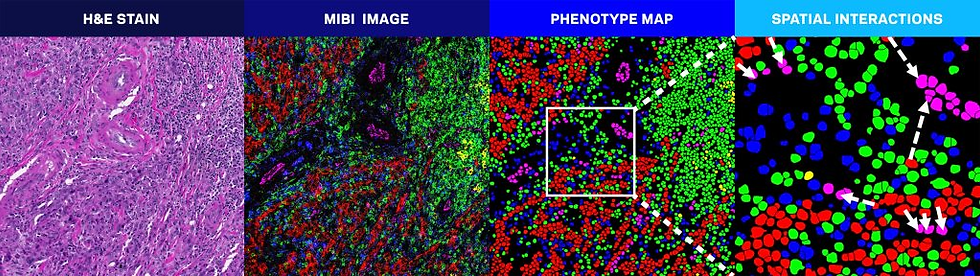
Although spatial biology tools are still a top priority in life-sciences research labs, clinical pathology laboratories have already seen a significant upstream benefit from them.
The tumor microenvironment (TME) plays a crucial role in cancer development and progression, and its characterization is critical for the development of effective therapies. The use of computer vision and AI in the analysis of the tumor microenvironment has enabled the identification of various biomarkers that have significant prognostic and predictive values. These biomarkers include immune cell infiltration, stromal composition, and tumor heterogeneity, among others. The use of these biomarkers has allowed for the precise identification of patient subgroups that are likely to benefit from specific treatments.

Unprecedented complexity in tissue-based biomarker expression and cellular organization is produced by multiplexing and spatial phenotyping. Our understanding of the nature of diseases is expanded by using quantitative digital pathology and artificial intelligence tools to manage this complexity.
Systems biology is also taking on new dimensions as a result of the definition and measurement of spatial distribution. Parallel to this, the facilitation of Macrophage migration inhibitory factor (MIF)by the quantification of expression (and co-expression) of multiple biomarkers at a cellular level opens a completely new route for developing predictive tests in oncology.
The gap between genomic/transcriptomic analysis and conventional histomorphology evaluation is filled by spatial biology. Here, we uncover the mechanisms underlying the communication between cancer cells or molecular phenotypes and their microenvironments. This knowledge will open new treatment options and point the way to new predictive biomarkers.
Challenges that lie ahead:
Analysis of biopsy slides is one of the most promising methods to capture the complexity of the TME and its function in drug response because TME information is spatial by nature. Millions of data points from whole-slide images could be extracted by computer vision algorithms to provide a detailed description of the tumor and TME and find the subtle immune signatures that distinguish responders from non-responders.
Nevertheless, with new onset of technology and navigating the stream of new application, there are various challenges that needs to be addressed:
Data analysis: One of the biggest challenges in spatial biology is the sheer volume of data that is generated. With the advent of new technologies such as single-cell sequencing and spatial transcriptomics, pathologists now have access to an unprecedented amount of data. However, analyzing and interpreting this data can be a daunting task. Pathologists must be skilled in computational analysis and have access to the appropriate software tools to make sense of the data.
Integration with existing pathology workflows: Spatial biology technologies are still relatively new, and there is a learning curve for pathologists who are not familiar with these tools. Additionally, integrating spatial biology data into existing pathology workflows can be challenging. Pathologists must be able to seamlessly integrate spatial data with traditional histopathology data, which requires specialized expertise.
Standardization: There is currently no standardization of spatial biology techniques, and different laboratories may use different protocols and methodologies. This can lead to variability in results and difficulties in comparing data across different studies. Pathologists must work to establish standardized protocols to ensure reproducibility and consistency in spatial biology research.
Validation of spatial data: Validating spatial data can be challenging due to the large amount of noise and technical variability that can arise during sample processing and data analysis. Pathologists must be able to validate spatial data to ensure that the results are accurate and reliable.
A Holistic Solution:
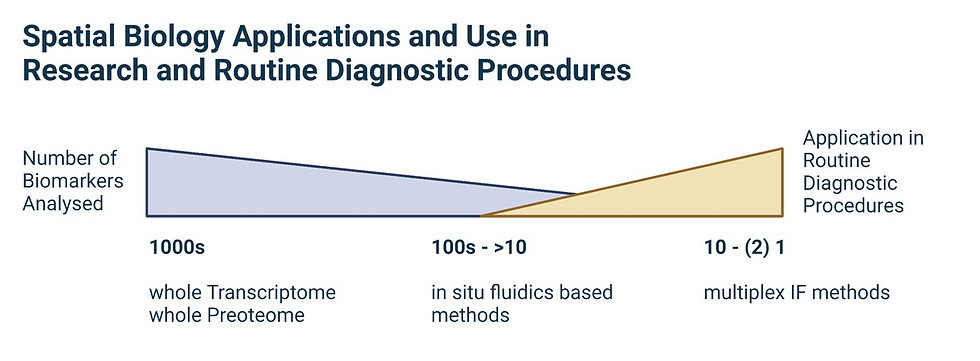
Building solutions that become the beacon of light for next generation of digital pathology and spatial biology is the top priority for PRR.
With holistic solutions that drive forward change and evolution features that assist for a better diagnosis and prognosis:
Visualizing & quantifying tissue micro-environment: essential for understanding the underlying biological processes that occur in a specific tissue with powerful visualization techniques, PRR helps you get insights into disease mechanisms and identify potential therapeutic targets, ultimately leading to improved patient outcomes.
Dynamic computer vision models & image analysis techniques: with the ability to process and analyze large amounts of image data and gain a deeper understanding of the cellular and molecular processes that occur within tissues our computer vision models assist you in gaining comprehensive view of disease processes.
Integrate data from multiple-omics technologies: The integration of data from genomics, transcriptomics, proteomics, metabolomics, and other omics technologies can provide a more comprehensive understanding of the molecular mechanisms involved in disease development and progression.
Connect with us on how you can explore the advancing technology to inculcate into your pathology workflow and build for a resilient future.
References:
E Berglund et al., “Automation of Spatial Transcriptomics library preparation to enable rapid and robust insights into spatial organization of tissues,” BMC Genomics, 21, 298 (2020). PMID: 32293264.
MV Hunter et al., “Spatially resolved transcriptomics reveals the architecture of the tumor-microenvironment interface,” Nat Common, 12, 6278 (2021). PMID: 34725363.
A Chen et al., “Spatiotemporal transcriptomic atlas of mouse organogenesis using DNA nanoball-patterned arrays,” Cell, 185, 1777 (2022). PMID: 35512705.
SM Lewis et al., “Spatial omics and multiplexed imaging to explore cancer biology,” Nat Methods, 18, 997 (2021) PMID: 34341583.
Comments